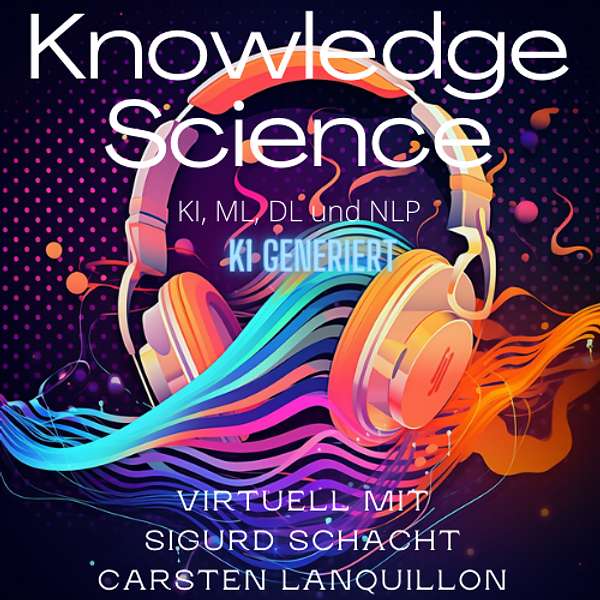
Knowledge Science - Alles über KI, ML und NLP
Knowledge Science - Alles über KI, ML und NLP
Episode 152 - English AI generated : KS Pulse - Phi-3, GARAG
English Version - The German Version also exists, but content differ minimal:
AI-generated News of the Day. The Pulse is an experiment to see if it is interesting to get the latest news in 5 min. small packages generated by an AI every day.
It is completely AI-generated. Only the content is curated. Carsten and I select suitable news items. Thereafter, both the manuscript and the audio file are created completely automatically.
Accordingly, we cannot always guarantee accuracy.
Topic 1: Phi-3 Technical Report: A Highly Capable Language Model Locally on Your Phone. https://arxiv.org/pdf/2404.14219.pdf
Topic 2: Typos that Broke the RAG’s Back: Genetic Attack on RAG Pipeline by Simulating Documents in the Wild via Low-level Perturbations https://arxiv.org/pdf/2404.13948
It would be great if you compare the German to the English version and give us feedback.
Hello listeners! This is Sigurd, your host for the "Knowledge Science Pulse" podcast, where we dive into the fascinating world of AI. Today, I'm joined by my co-host Carsten, and we'll be discussing two exciting academic papers that explore the capabilities and robustness of language models.
#### Greetings, Sigurd! I'm thrilled to be here and discuss these groundbreaking papers. Let's start with the first one, "A Highly Capable Language Model Locally on Your Phone" by Microsoft. What caught my attention is the introduction of phi-3-mini, a language model with 3.8 billion parameters that can run on a phone yet rivals the performance of models like GPT-3.5 and Mistral 8x7B.
#### Absolutely, Carsten! Imagine having a supercomputer's capabilities on your smartphone. The innovation lies in the training data, composed of heavily filtered web data and synthetic data. By optimizing the data for reasoning ability, the researchers achieved remarkable performance on benchmarks like MMLU and MT-Bench, despite the model's compact size.
#### Precisely, Sigurd! And what's even more impressive is that they've taken this approach further by introducing phi-3-small and phi-3-medium, larger models with 7 billion and 14 billion parameters, respectively. These models demonstrate a significant improvement over phi-3-mini, achieving scores as high as 78% on MMLU and 8.9 on MT-Bench.
#### That's incredible, Carsten! It's fascinating to see how optimizing the training data can lead to such impressive performance gains, even in smaller models. But tell me, how did they ensure the robustness and safety of these language models?
#### An excellent question, Sigurd! The researchers employed a multi-pronged approach, including post-training with instruction fine-tuning and preference tuning using datasets focused on helpfulness and harmlessness. They also conducted extensive testing and evaluations across various RAI harm categories, iteratively refining the models based on feedback from a dedicated red team.
#### That's commendable, Carsten. Ensuring the responsible development of AI systems is crucial, and it's reassuring to see the rigorous measures taken by the researchers. Now, let's move on to the second paper, "Typos that Broke the RAG's Back: Genetic Attack on RAG Pipeline by Simulating Documents in the Wild via Low-level Perturbations."
#### Ah yes, Sigurd, this paper explores a fascinating and often overlooked aspect of language model robustness – vulnerability to noisy documents containing minor textual errors. The authors introduce GARAG, a novel adversarial attack method that targets both the retriever and reader components of Retrieval-Augmented Generation (RAG) systems.
#### Absolutely, Carsten! The researchers identified a critical gap in existing studies, which often focus on either the retriever or the reader component in isolation. By considering the sequential interaction between these components, they uncovered a significant vulnerability in RAG systems when faced with low-level perturbations like typos, punctuation errors, and character swaps.
#### Precisely, Sigurd! The results are quite alarming. GARAG consistently achieved high attack success rates, around 70%, across various retriever and language model combinations. Moreover, the end-to-end performance of the RAG systems suffered significant degradation, with exact match scores dropping by up to 50% in some cases.
#### Those are indeed concerning findings, Carsten. It highlights the importance of considering real-world scenarios when evaluating the robustness of AI systems. Minor textual errors, which are prevalent in databases and online sources, can potentially disrupt the entire RAG pipeline, leading to incorrect or unreliable outputs.
#### Absolutely, Sigurd. And what's even more worrying is that lower perturbation rates seemed to pose a greater threat to the RAG system. This emphasizes the need for robust techniques to mitigate these inconspicuous yet critical vulnerabilities, as even a few typos can have a substantial impact on the system's performance.
#### You raise an excellent point, Carsten. These findings underscore the importance of developing robust and resilient language models that can handle noisy and imperfect data, which is often the reality in real-world scenarios. Researchers and developers must prioritize these aspects to ensure the safe and reliable deployment of AI systems.
#### Agreed, Sigurd. As we continue to push the boundaries of AI capabilities, it is crucial to address these vulnerabilities proactively. The insights from these papers will undoubtedly contribute to the development of more robust and trustworthy language models, capable of handling the complexities of real-world data while maintaining high performance and reliability.
#### Well said, Carsten. I believe our listeners have gained valuable insights into the cutting-edge research happening in the field of language models. Thank you for this engaging discussion, and to our listeners, stay tuned for more exciting episodes of "Knowledge Science Pulse," where we explore the latest developments in AI and their implications for the future.